While in traditional software a programmer implements fixed rules for the program, the AI derives these decision rules itself: Here, the computer uses learning methods or algorithms to derive statistical regularities or patterns from existing data. These patterns can be represented by different models and allow us to make accurate predictions for new data. However, for a variety of AI applications, the learned decision rules of established AI technologies are hard to understand.
Compared to the traditional program where the decision rules are predefined and therefore transparent and comprehensible for the programmer, many of the established AI technologies still represent a „black box“: It is often neither comprehensible nor transparent how the system arrives at decisions or results.
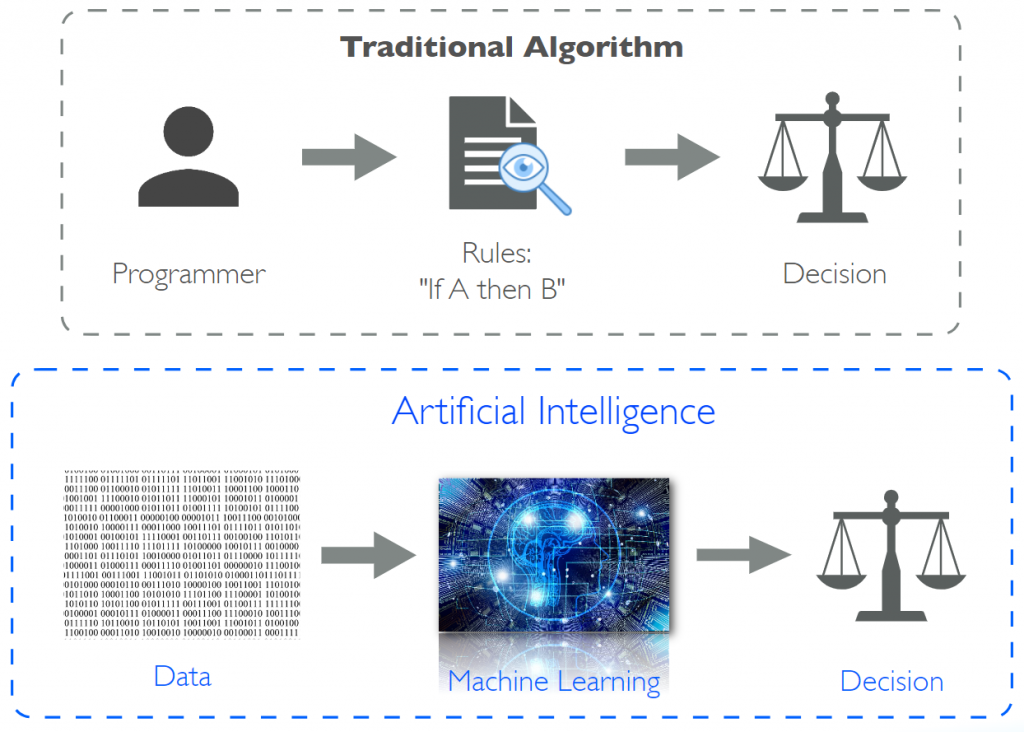